AI
6 Things AI and Machine Learning Reshape in SW
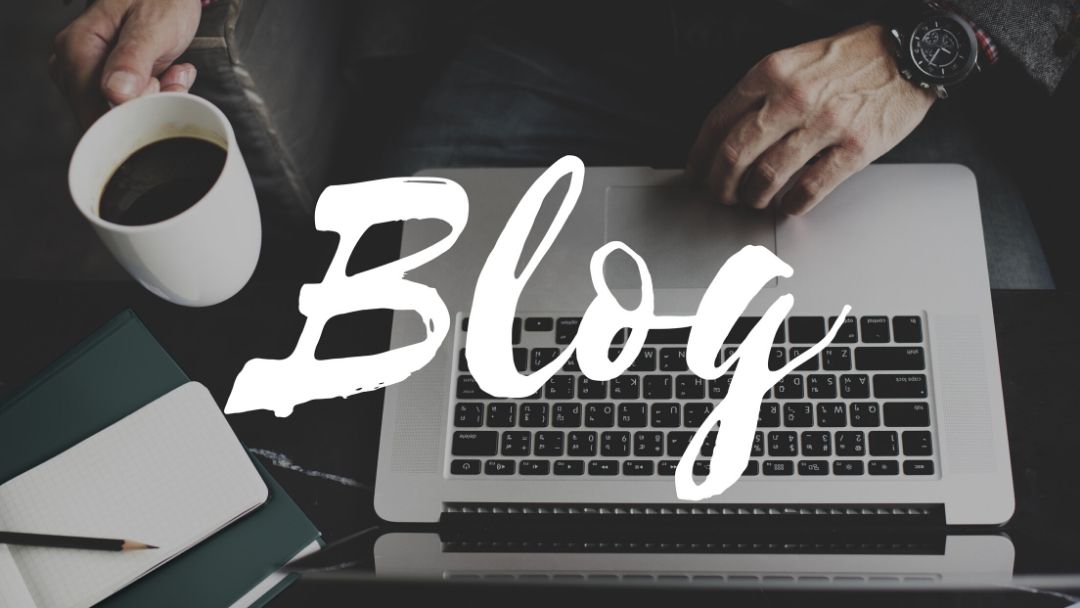
In my recent post, I mentioned that AI and ML challenge architecture, but also offer tools to tackle the challenges. Needless to say, automation of repetitive tasks will change our job descriptions, just like those of our end users.
The SW development lifecycle is changing too. Architects with experience from an environment with external content prosumers or open-source developers, will find some lifecycle changes similar to those two environments.
1. Crowd management
Much of the “crowd” involved is outside the IT organization (e.g. experts in domains other than IT), or even outside the enterprise – not least in Data as a Service offering ML from data sources such as digital twins (of customer-owned equipment, e.g. railroads and trains at Siemens, networks at Ericsson, or farming machinery at John Deere). Architects or CIOs have influence rather than full control, unlike in internal projects.
2. Crowdsourcing
Both the data and some ML-generated logic come from external sources, not least when some ML and computing runs locally on “edge” devices that produce the input data.
3. Adaptive planning
Distinct project phases tend to disappear, partly because of the “crowd” out there, partly because of the explorative nature of ML (“think more like a researcher, less like a programmer”). For example, a partial result of an ML project can hint about additional key domains to drill into, thus widening the scope and postponing the deadline.
4. Incomplete requirements
Customers may have a rather sketchy idea of what they want. “More bang for the buck” wouldn’t hint on “increased harvest, better soils, 80 percent less herbicides due to spraying individual weed plants only”, but ML with fast pattern recognition (in RT field images) does exactly that.
5. Widened job roles
Apart from the SW (development) lifecycle and the nature of new apps, AI reshapes the way they’re developed and thus our roles too. Architects and some devs become even curators of training data sets, co-analysts of ML results, and a guide for experts from non-IT domains, to enable them to apply ML in their specific tasks.
6. A hard core (platform)
The core (e.g. an automated data/ML platform) has to be secure, robust, modular, reliable (fault-tolerant, even on external error), documented and teachable to teams within the enterprise. The ML-generated system has to interoperate with other, programmer-made, systems (pre-ML AI, and other SW). The ML-generated logic has to be auditable and verifiable; indeed, explainability is the door to acceptance in mission-critical apps.
Figure from course AI, Architecture, and Machine Learning
3 kommentar/er till “6 Things AI and Machine Learning Reshape in SW”
Kommentarer är inaktiverade.
Lämna en kommentar
I like your post very much. It is very much useful for my research. I hope you to share more info about this. Keep posting artificial intelligence online course
Thanks for sharing this valuable information and we collected some information from this blog.
Artificial Intelligence Training in Noida
Good Post. I like your blog. Thanks for Sharing.
Machine Learning Training in Noida