AI
Yet another AI language you miss in your CV? 4 reasons why it will matter less and less.
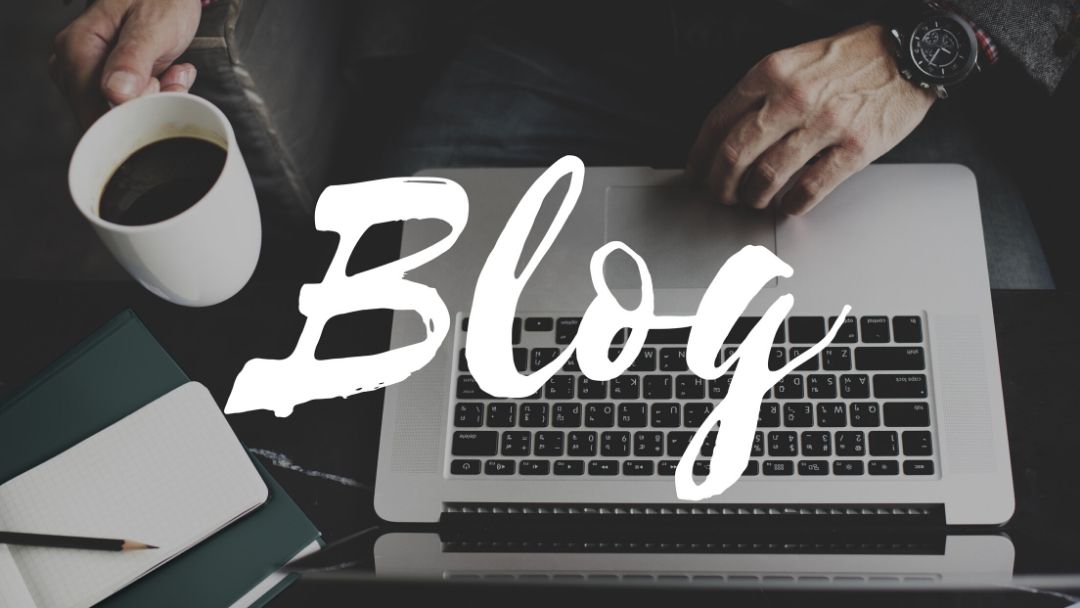
It never hurts, but it varies how helpful a (fairly) new programming or script language is. From more or less a prerequisite in R&D and platform-vendor firms, to a nice-to-have CV footnote in mainstream businesses that rather emphasize extended SQL, analytics, data architecture, and automated ML platform/s.
Here are 4 reasonswhy you can live with it (ascending order by weight)
1. The fate of LISP, Prolog, Smalltalk, KQLM etc. (use comment form below to fill in what’s missing : ))
To drive the history of applied “AI 1.0” to the extremes, an enterprise in the 1980-ies was expected to achieve superpowers as soon as the CIO (and preferably, CEO : )) learned at least one exotic-enough AI language. The subsequent AI winter after 1990 happened supposedly because most CIO’s refused to so; best case: they got lost somewhere inside their fifth pair of nested parentheses in LISP (like most of us devs did too, including myself)…
2. The rise of expert-system development shells in business (near 1990)
Programming languages are versatile. You can get anything you need, given a generous timeframe. Development tools encapsulate a lot of technical detail. You can get more or less what you need, even under tight time constraints. No wonder it’s more appealing to CIOs than nested parentheses.
3. Success of those who used then-mainstream industry languages
Books on configurators, or on LISP, drill down into Digital (HP) XCON/R1, but in books on management and modular manufacturing, you read about Scania Trucks & Buses (reporting profits for 80 consecutive years). Scania’s smart proprietary configurator (an age fellow of XCON) became a backbone of the enterprise, and grew to several times the size of XCON. Unlike XCON, Scania did cope in maintenance and upgrades. For decades. Offering complex customizedvehicles assembled from a cluster of common component types. Language: unglamorous then-mainstream Cobol and DL/1.
4. A trend toward frameworks, component libraries, automated analytics & ML platforms
It’s a two-way street. On one hand, “AI 2.0” and ML challenge current architectures, not least data architectures: big-data ingestion, parallelism, fast access aid for non-sequential access because learning (in both human and artificial neural networks) is essentially non-sequential (parallel).
On the other hand, ML offers a toolbox to tackle these challenges, and also, enables quite some automation of the entire data pipeline and of an architect’s (or dev’s) repetitive tasks. I won’t be surprised if automated-ML platforms for big data, using extended SQL instead of script languages, spark success stories of Scania’s magnitude. It’s about the augmentation (or automation) itself, and about architecture fit for business, rather than about the detail.
So from now on, Informator’s new one-day course is called AI, Architecture, and Machine Learning. Neither just AI for Architecture, nor just Architecture for AI. It’s a two-way street.
Rapid progress in the middle
Figure from course AI, Architecture, and Machine Learning (T1913)